As industries like construction, urban planning, and environmental monitoring continue to adopt advanced technologies such as LiDAR (Light Detection and Ranging) and photogrammetry the use of point clouds has become a critical tool for capturing real-world environments in 3D. However, the raw data collected through point clouds can be overwhelming and complex. This is where point cloud classification becomes essential, transforming unstructured 3D data into actionable insights.
In this article, we will explore why you should classify your point clouds, the advantages of classified point clouds over unclassified ones, key use cases, and how you can utilise artificial intelligence (AI) for the classification process.
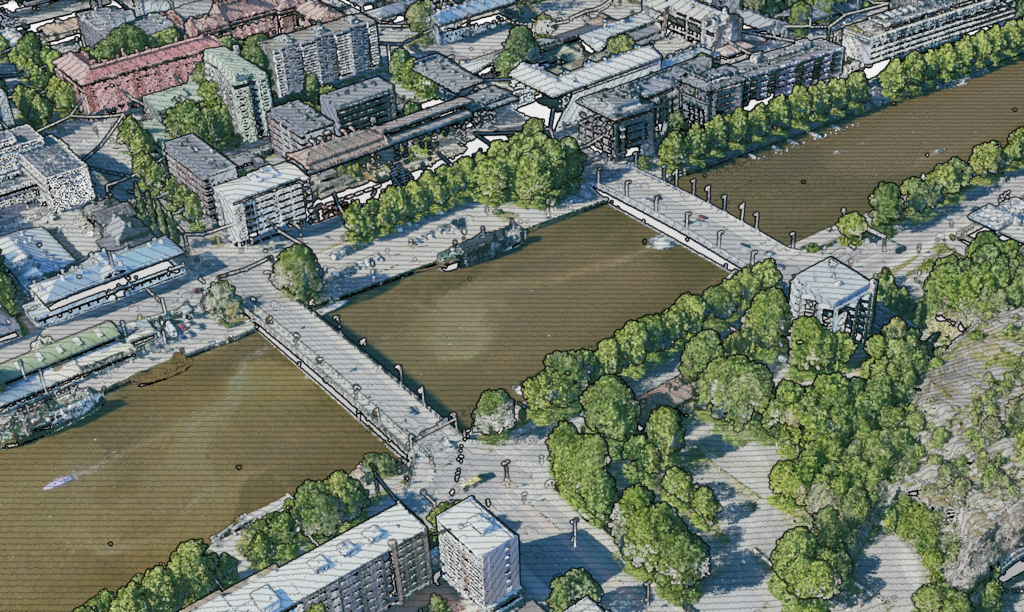
Why Classify Point Clouds?
Point clouds are rich, detailed datasets that are collections of data points representing the surface geometry of physical objects. Without classification, point clouds are just a collection of unorganised points, as raw point clouds are often vast and unorganised, containing millions or even billions of data points. They remain difficult to interpret and analyse without classification. When you classify a point cloud, you can segment the data into categories—such as ground, vegetation, or infrastructure—unlocking its true potential.
Here are some of the key reasons why you should prioritise point cloud classification:
- Improved Data Usability: Classification turns raw data into categorized groups, such as ground, vegetation, buildings, and infrastructure. This segmentation makes it easier for businesses to interpret the data and make informed decisions.
- Enhanced Precision: Classified point clouds allow for more accurate measurements and modeling of specific features. For example, identifying roads, bridges, or building facades separately from surrounding terrain reduces error in project planning and analysis.
- Better Resource Management: By focusing on specific categories (e.g., isolating power lines in a utility survey), businesses can optimize resources for targeted analysis, reducing time and cost.
- Automation of Workflows: Classification supports automated workflows, especially in industries like construction and urban planning, where different asset types require different treatments.
- Regulatory Compliance: For industries like mining, forestry, or environmental monitoring, classified point clouds can help ensure compliance with regulations by providing detailed and accurate reports on land use or natural resources.
Classified vs. Unclassified Point Clouds: Key Differences and Use Cases
When you compare classified and unclassified point clouds, the key difference is how easily the data can be understood and applied to specific use cases.
Unclassified Point Clouds
Unclassified point clouds are raw datasets that consist of millions of points representing the surfaces of objects but without any structure or categorisation. While they offer a comprehensive, highly detailed representation of the scanned environment, the data is difficult to navigate and analyse without significant manual effort.
Use Cases for Unclassified Point Clouds:
- Initial Data Capture: In the early stages of data collection, such as during a drone or LiDAR scan, unclassified point clouds are crucial for capturing the entirety of a scene without pre-filtering the data.
- 3D Modeling and Rendering: For applications focused purely on visualization—like creating 3D models for entertainment or architectural renderings—unclassified point clouds may be sufficient.
- Exploratory Research: In cases where the point cloud will be examined manually or without the need for segmentation, such as in scientific research, raw, unclassified data can be used for initial exploration.
Classified Point Clouds
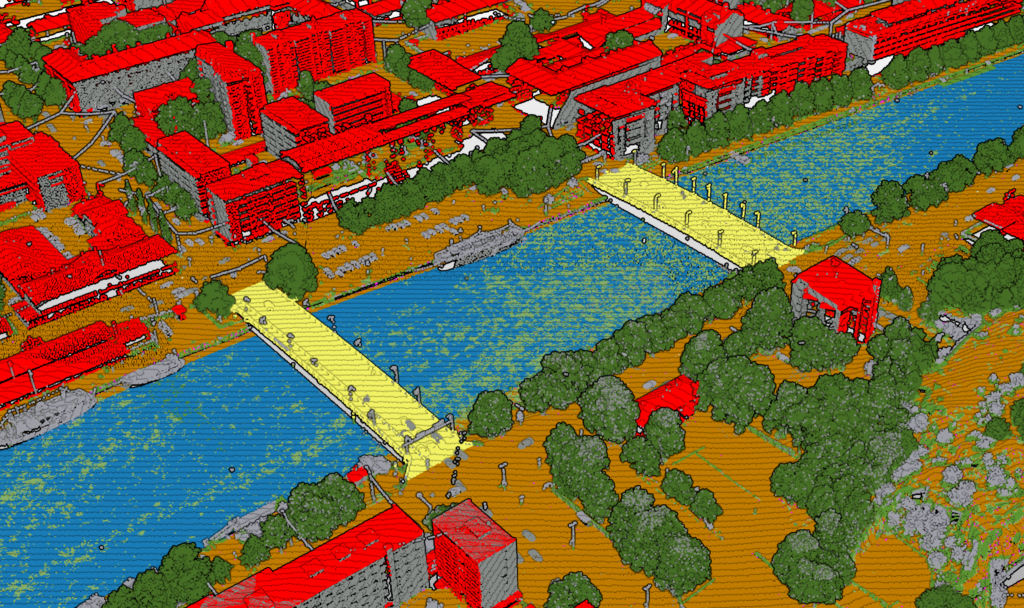
Classified point clouds transform raw, unstructured data into organised, actionable categories. By labeling points into predefined groups—such as ground, buildings, vegetation, or infrastructure—this process enables specific analysis and easier data manipulation. For example, on a construction site, classified data can separate buildings from roads or terrain, providing clarity and precision for decision-making.
Use Cases for Classified Point Clouds:
- Urban Planning and Infrastructure Development: By distinguishing between roads, buildings, and open spaces, classified point clouds give planners the data they need to make informed decisions about infrastructure expansion and urban zoning. For example, the City of Helsinki used classified point clouds to create a detailed 3D city model. This model helped optimize urban planning and infrastructure management, making the process more efficient and accurate. Read more about this: Helsinki 3D
- Surveying and Mapping: They enable you to isolate ground points from vegetation or structures, simplifying accurate topographic analysis and facilitating the creation of detailed Digital Elevation Models (DEMs).
- Forestry and Environmental Monitoring: Classification helps you monitor tree canopy density, assess forest health, and track land degradation while filtering out non-natural elements. For example, researchers utilized classified LiDAR point clouds to measure forest canopy structure and biomass across Amazonian landscapes. This approach has been instrumental in monitoring the effects of selective logging on forest biomass and carbon balance, as well as understanding forest recovery over time. Learn more from Arxiv.
- Utility Management: For utility companies, classifying point clouds enables the identification of power lines, poles, and surrounding vegetation, helping to improve maintenance operations and risk assessment.
The Process of Point Cloud Classification
Point cloud classification organises millions of data points into meaningful categories, leveraging a combination of manual effort, algorithms, and AI-driven automation.
- Preprocessing: Point clouds often undergo preprocessing steps to clean and filter the data before classification. This might involve removing noise, outliers, or redundant points, ensuring that only relevant data is classified.
- Manual Classification: In traditional workflows, classification was done manually by human operators who would segment the point cloud based on visual inspection and known features. However, this process is labour-intensive, time-consuming, and prone to human error, making it impractical for large datasets.
- Algorithmic Classification: Many modern point cloud processing tools include rule-based algorithms to classify points automatically. For example, algorithms can distinguish between ground points and non-ground points using height and slope thresholds. While this speeds up the process, it may still require human supervision and adjustments.
- Machine Learning and AI: Today, artificial intelligence and machine learning (ML) models are revolutionising point cloud classification. AI models can be trained on large datasets to recognise patterns and features in point clouds, allowing them to classify points into specific categories automatically and with high accuracy. This reduces manual effort and improves the speed and precision of classification.
The Role of AI in Point Cloud Classification
Artificial intelligence is transforming point cloud classification by delivering unmatched speed, accuracy, and scalability. By leveraging advanced technologies like deep learning, AI can efficiently organise and analyse complex 3D data. Models such as convolutional neural networks (CNNs) and point-based networks enable AI to recognise and categorise features within a point cloud, distinguishing between elements like ground, trees, and man-made structures.
AI excels at feature recognition, identifying objects based on their geometry, texture, and spatial relationships. For example, it can differentiate roads, buildings, and vegetation by analysing their shape, size, and positioning. Additionally, AI systems continuously learn and improve over time. As they process and label more point clouds, their accuracy increases, significantly reducing the need for manual input.
One of AI’s standout advantages is scalability. In industries like city planning, mining, or infrastructure development, where massive datasets are common, AI can handle large volumes of data far faster than manual processing, enabling more efficient workflows and better decision-making.
Why Classified Point Clouds Matter
Classified point clouds are the key to getting the most out of your 3D data. Whether you’re in urban planning, surveying, or another industry, they help you work smarter, not harder. Thanks to AI and machine learning advancements, classification is now faster and more accurate than ever—giving you the insights you need to make informed decisions, optimise workflows, and boost efficiency.
With Pointscene, you can take your classified data even further. Visualise, share, and analyse it seamlessly to unlock new possibilities for your projects. Ready to transform how you work with 3D data? Head to Pointscene.com to learn more.
Conclusion Point cloud classification is a transformative process that turns vast, unstructured datasets into actionable insights. Classification enhances usability, accuracy, and efficiency across industries like urban planning, construction, forestry, and utility management by categorising data into meaningful groups. It enables businesses to streamline workflows, save time, and make more informed decisions.
Artificial intelligence and machine learning advancements have revolutionised the classification process, making it faster, more precise, and scalable. By automating the labour-intensive aspects of classification, AI allows companies to process massive datasets efficiently, improve decision-making, and reduce costs.
With tools like Pointscene, classified point clouds can go even further. By enabling real-time visualisation, seamless sharing, and in-depth analysis, Pointscene helps businesses extract maximum value from their 3D data. Classified point clouds are no longer just data—they are powerful assets driving smarter operations and better outcomes.
You can read more articles like this on our blog: Pointscene.com
Some of our top pics:
Revolutionizing Point Cloud Data Collection: Handheld iPhone Scanners with Enhanced GPS
Construction Site Mapping with Drones: RTK vs. Traditional Methods